Comprehensive Guide To Data Annotation Tech Assessment Answers: Tips, Techniques, And Insights
In today’s data-driven world, data annotation has emerged as a critical component of machine learning and artificial intelligence systems. From autonomous vehicles to natural language processing, data annotation tech assessment answers play a pivotal role in ensuring the accuracy and reliability of AI models. But what exactly does data annotation entail, and how can one master the skills required to excel in this field? This article dives deep into the subject, providing a detailed roadmap for understanding and excelling in data annotation assessments. Whether you’re a beginner or an experienced professional, this guide will equip you with actionable insights and strategies to ace your next tech assessment.
As organizations increasingly rely on AI and machine learning, the demand for high-quality annotated data has skyrocketed. Data annotation tech assessment answers are not just about labeling data correctly; they also test your ability to understand complex instructions, adhere to quality standards, and meet deadlines. In this article, we’ll explore the nuances of data annotation, discuss its importance in AI development, and provide practical tips to help you succeed. By the end of this guide, you’ll have a comprehensive understanding of data annotation assessments and how to approach them effectively.
From understanding the various types of data annotation to mastering the tools and techniques used in the process, this article will serve as your ultimate resource. We’ll also answer common questions about data annotation tech assessment answers, provide expert advice, and share insights from credible sources. So, buckle up and get ready to delve into the fascinating world of data annotation!
Read also:Discovering The Impact Of Fox Lisa Boothe A Comprehensive Guide
Table of Contents
- What is Data Annotation and Why Does It Matter?
- What Are the Different Types of Data Annotation?
- What Tools Are Essential for Data Annotation?
- How to Master Data Annotation Techniques?
- What Are the Common Challenges in Data Annotation?
- How to Prepare for Data Annotation Tech Assessment Answers?
- Real-World Applications of Data Annotation
- Frequently Asked Questions About Data Annotation Tech Assessment Answers
What is Data Annotation and Why Does It Matter?
Data annotation is the process of labeling raw data—such as text, images, audio, or video—to make it understandable for machine learning algorithms. This process is crucial because AI models cannot interpret raw data without proper labeling. For instance, in an image recognition task, data annotation involves tagging objects within the image, such as cars, pedestrians, or traffic lights. Similarly, in natural language processing, data annotation involves labeling parts of speech, sentiment, or named entities in a text.
Why does data annotation matter? The quality of annotated data directly impacts the performance of AI models. Poorly annotated data can lead to inaccurate predictions, which can have serious consequences in fields like healthcare, autonomous driving, and cybersecurity. Data annotation tech assessment answers are designed to evaluate your ability to produce high-quality annotations consistently. This is why mastering data annotation is not just a skill but a necessity for anyone aspiring to work in AI and machine learning.
Why Is High-Quality Data Annotation Critical for AI Models?
High-quality data annotation ensures that AI models can learn effectively from the data they are trained on. Here’s why it’s so critical:
- Accuracy: Properly annotated data reduces errors and improves the model’s ability to make accurate predictions.
- Efficiency: High-quality annotations speed up the training process, saving time and resources.
- Reliability: Well-annotated data ensures that the AI model performs consistently across different scenarios.
How Does Data Annotation Impact Real-World Applications?
Consider the example of autonomous vehicles. These vehicles rely on annotated data to identify objects like pedestrians, road signs, and other vehicles. If the annotations are incorrect, the AI system may fail to recognize a pedestrian, leading to potentially catastrophic outcomes. Similarly, in healthcare, annotated medical images help AI systems diagnose diseases accurately. The stakes are high, and the importance of data annotation cannot be overstated.
What Are the Different Types of Data Annotation?
Data annotation can be broadly categorized into several types, each tailored to specific use cases and data formats. Understanding these types is essential for anyone preparing for data annotation tech assessment answers.
Text Annotation
Text annotation involves labeling text data to make it interpretable for natural language processing (NLP) models. Common types of text annotation include:
Read also:Unblocked Games Your Ultimate Guide To Fun And Learning
- Named Entity Recognition (NER): Identifying and labeling entities like names, dates, and locations in a text.
- Sentiment Analysis: Labeling text based on its emotional tone (positive, negative, or neutral).
- Part-of-Speech Tagging: Labeling words based on their grammatical roles (nouns, verbs, adjectives, etc.).
Image Annotation
Image annotation involves labeling objects, regions, or features within an image. This type of annotation is widely used in computer vision applications. Key techniques include:
- Bounding Boxes: Drawing rectangles around objects of interest.
- Polygon Annotation: Labeling irregularly shaped objects with polygons.
- Pixel-Level Annotation: Labeling every pixel in an image for tasks like semantic segmentation.
How Does Audio Annotation Differ from Other Types?
Audio annotation involves labeling audio files for tasks like speech recognition, emotion detection, or speaker identification. Unlike text and image annotation, audio annotation requires specialized tools and expertise to handle nuances like background noise and accents.
What Tools Are Essential for Data Annotation?
To excel in data annotation tech assessment answers, you need to be familiar with the tools commonly used in the industry. These tools streamline the annotation process and ensure consistency and accuracy.
Popular Data Annotation Tools
Here are some of the most widely used data annotation tools:
- Labelbox: A versatile platform for image, text, and video annotation.
- Supervisely: Known for its advanced features in image and video annotation.
- Prodigy: A powerful tool for NLP tasks like text classification and named entity recognition.
How to Choose the Right Tool for Your Needs?
Choosing the right tool depends on factors like the type of data you’re working with, the complexity of the task, and your budget. For instance, if you’re working on a large-scale image annotation project, a tool like Supervisely might be more suitable. On the other hand, if you’re focusing on text annotation, Prodigy could be a better fit.
How to Master Data Annotation Techniques?
Mastering data annotation techniques is essential for excelling in data annotation tech assessment answers. Here are some tips to help you improve your skills:
Develop a Systematic Approach
Creating a step-by-step workflow can help you stay organized and efficient. For example:
- Review the annotation guidelines thoroughly.
- Start with a small sample to ensure you understand the task.
- Use keyboard shortcuts and other features to speed up the process.
What Are Some Common Pitfalls to Avoid?
Here are some common mistakes to watch out for:
- Inconsistent Labeling: Ensure that your annotations are consistent across the dataset.
- Overlooking Edge Cases: Pay attention to rare or ambiguous cases that might confuse the AI model.
- Ignoring Quality Checks: Always double-check your work to maintain high standards.
What Are the Common Challenges in Data Annotation?
Despite its importance, data annotation is not without its challenges. Understanding these challenges can help you prepare better for data annotation tech assessment answers.
Data Quality Issues
Poor-quality data, such as blurry images or noisy audio, can make annotation difficult. It’s essential to flag such issues early and communicate them to the relevant stakeholders.
How to Handle Ambiguity in Annotation Guidelines?
Ambiguous guidelines can lead to inconsistent annotations. If you encounter unclear instructions, don’t hesitate to seek clarification from your supervisor or team.
How to Prepare for Data Annotation Tech Assessment Answers?
Preparing for a data annotation tech assessment requires a combination of technical knowledge, practical experience, and strategic planning. Here are some tips to help you succeed:
Practice with Real-World Datasets
Working on real-world datasets can give you hands-on experience and help you identify areas for improvement. Platforms like Kaggle offer a wide range of datasets for practice.
What Are Some Effective Study Strategies?
Here are some strategies to consider:
- Break down complex tasks into smaller, manageable steps.
- Use online tutorials and courses to learn new tools and techniques.
- Join online communities to connect with other data annotators and share insights.
Real-World Applications of Data Annotation
Data annotation has a wide range of applications across various industries. Here are a few examples:
- Healthcare: Annotated medical images help AI systems diagnose diseases like cancer and pneumonia.
- Retail: Product recommendations are powered by annotated data from customer reviews and purchase histories.
- Autonomous Vehicles: Annotated images and videos enable self-driving cars to navigate safely.
Frequently Asked Questions About Data Annotation Tech Assessment Answers
What Skills Are Required to Excel in Data Annotation?
To excel in data annotation, you need a combination of technical skills (like familiarity with annotation tools) and soft skills (like attention to detail and problem-solving).
How Long Does It Take to Become Proficient in Data Annotation?
The time it takes to become proficient varies depending on your background and the complexity of the tasks. With consistent practice, most people can achieve proficiency within a few months.
Where Can I Find Resources to Improve My Data Annotation Skills?
Online platforms like Coursera, Udemy, and LinkedIn Learning offer courses on data annotation and related topics. Additionally, joining forums and communities can provide valuable insights and support.
Conclusion
Data annotation tech assessment answers are a gateway to a rewarding career in AI and machine learning. By understanding the fundamentals, mastering the tools and techniques, and staying updated on industry trends, you can position yourself as a skilled and reliable data annotator. Remember, the key to success lies in practice, persistence, and a willingness to learn. So, take the first step today and embark on your journey to mastering data annotation!
For further reading, check out this comprehensive guide on data annotation.
Unlock Premium Fare Deals Flyertalk: Your Ultimate Guide To Luxury Air Travel
Leonardo DiCaprio Net Worth 2023: A Deep Dive Into His Wealth And Career
What Does "CTFU Meaning Twitter" Really Signify? Explained
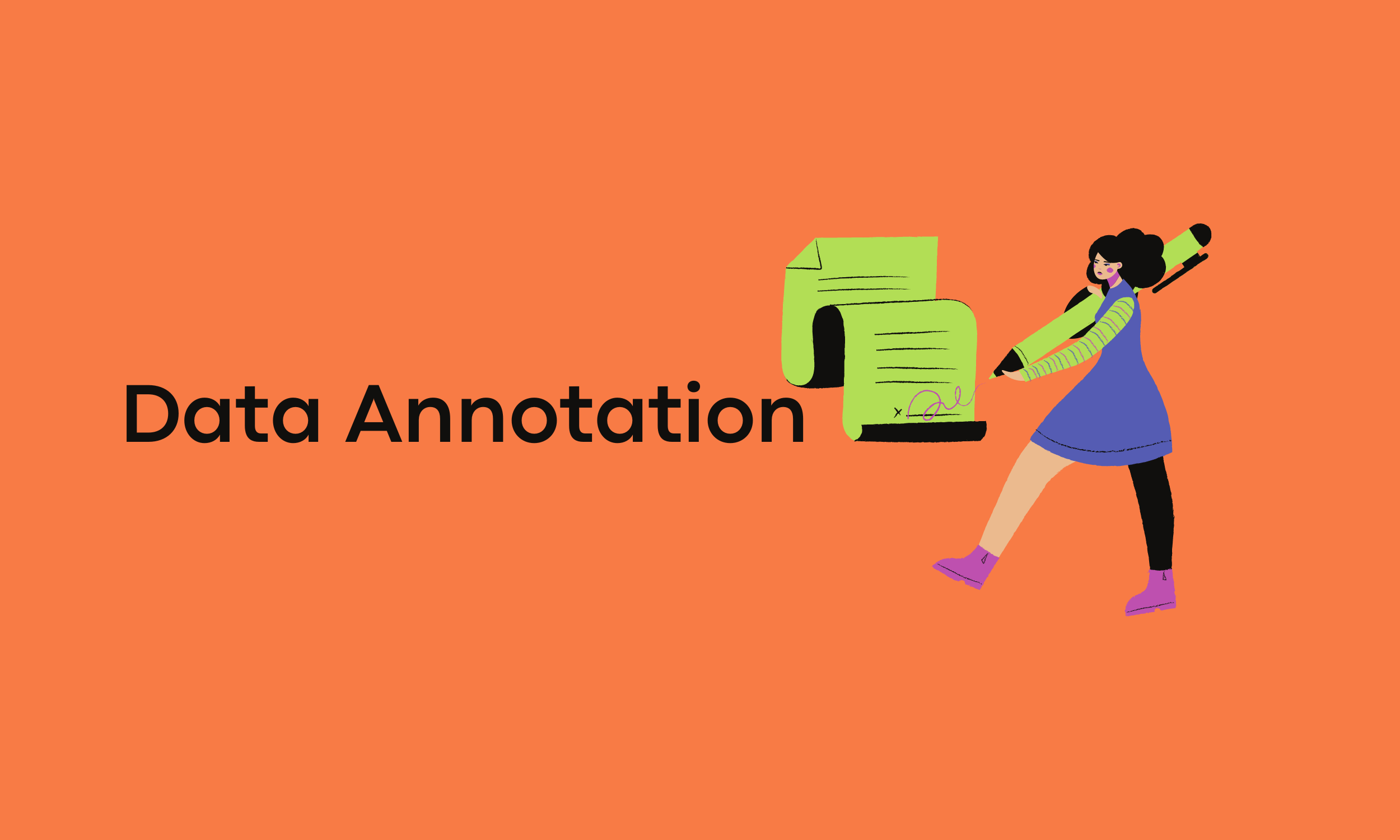
Data Annotation Odetta's spotlight — Odetta
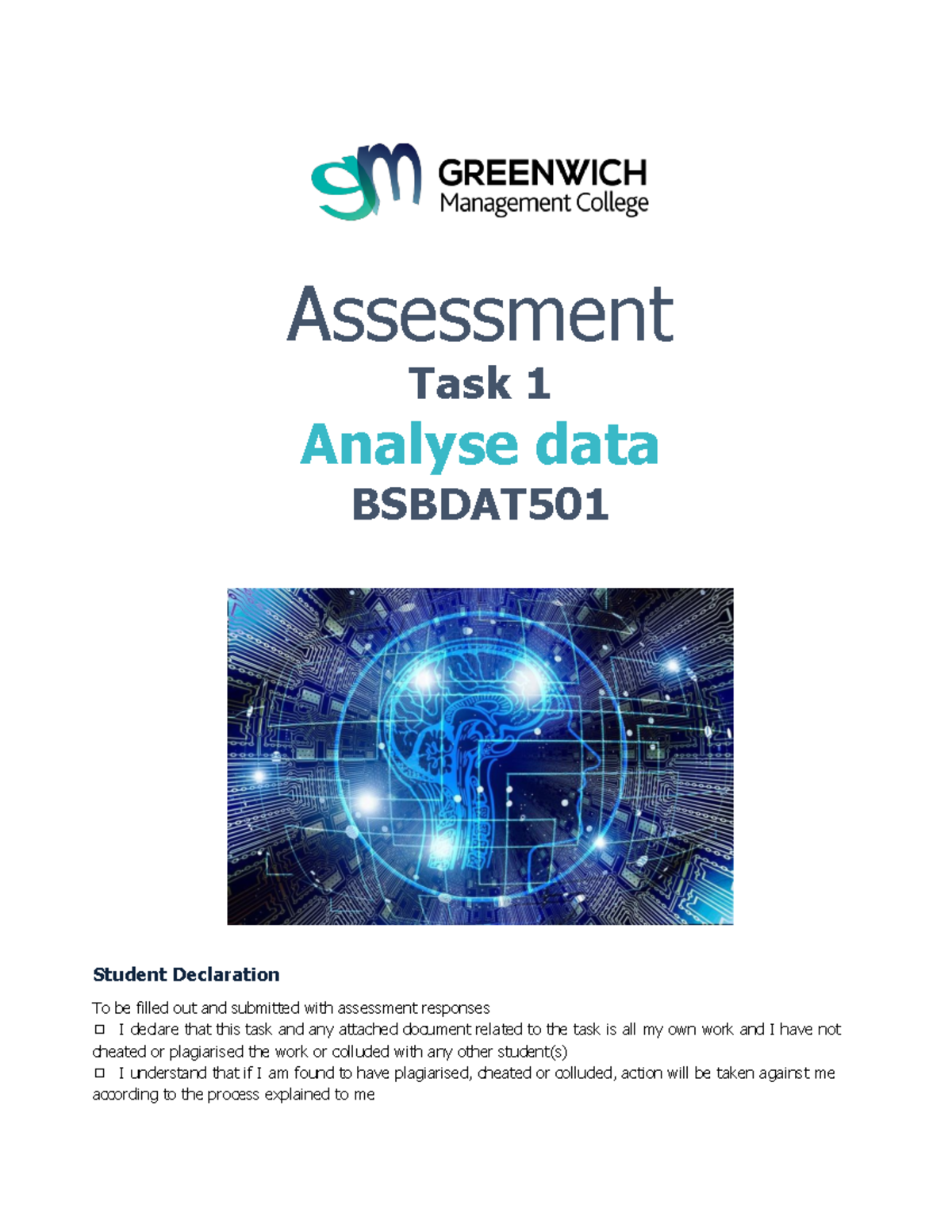
Data Annotation Starter Assessment Answers Intro And Short T